E Alwadee, X Sun, Y Qin, FC Langbein. Assessing and Enhancing the Robustness of Brain Tumor Segmentation using a Probabilistic Deep Learning Architecture. Proc ISMRM and ISMRT Annual Meeting and Exhibition, Singapore, May 2024.
[PDF]
[Slides:PDF]
[Video]
Motivated by the challenge of enhancing the robustness of deep neural network decisions against variable noise in MRI-based brain tumor segmentation, this study aims to evaluate the efficacy of probabilistic bottlenecks. Our approach simulates structured perturbations at increasing strength to assess their impact on segmentation performance utilizing the Wasserstein distance between per-sample Dice score distributions and the sensitivity with respect to the perturbation strength. Results show probabilistic bottlenecks significantly increase robustness to Gaussian noise, yet offer limited improvement towards Gaussian blur, with varying results for other perturbations, highlighting the perturbation-specific nature of network resilience.
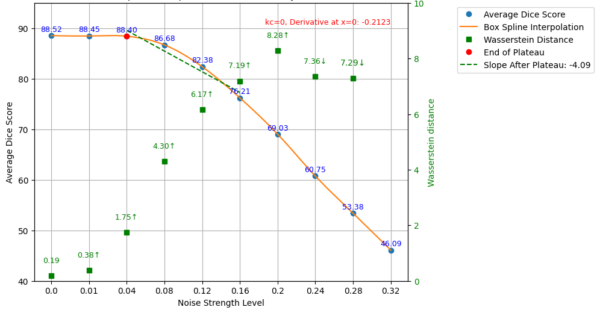
Robustness with respect to Gaussian noise
This work is licensed under a Creative Commons Attribution-NonCommercial-ShareAlike 4.0 International License.