I. Khalid, C. A. Weidner, E. A. Jonckheere, S. G. Schirmer, F. C. Langbein. Reinforcement Learning vs. Gradient-Based Optimisation for Robust Energy Landscape Control of Spin-1/2 Quantum Networks. IEEE Conf Decision and Control, pp. 4133-4139, 2021. [DOI:10.1109/CDC45484.2021.9683463] [arXiv:2109.07226] [PDF]
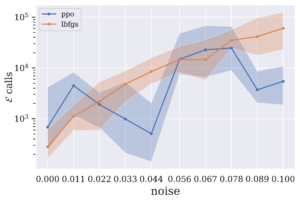
Number of environment calls comparison between L-BFGS and PPO for |0> to |2> for a chain of length 4 as a function of Hamiltonian perturbation noise.
We explore the use of policy gradient methods in reinforcement learning for quantum control via energy landscape shaping of XX-Heisenberg spin chains in a model agnostic fashion. Their performance is compared to finding controllers using gradient-based L-BFGS optimisation with restarts, with full access to an analytical model. Hamiltonian noise and coarse-graining of fidelity measurements are considered. Reinforcement learning is able to tackle challenging, noisy quantum control problems where L-BFGS optimization algorithms struggle to perform well. Robustness analysis under different levels of Hamiltonian noise indicates that controllers found by reinforcement learning appear to be less affected by noise than those found with L-BFGS.
This work is licensed under a Creative Commons Attribution-NonCommercial-ShareAlike 4.0 International License.